Krati Agarwal is a highly skilled data scientist and artificial intelligence expert with a passion for solving complex problems through mathematical concepts and programming. With three years of experience in the field, Krati has honed her skills through a combination of education and hands-on experience. In this interview, Krati shares her journey as to how she structured her education to meet her career goals, and what a typical day looks like as a consultant data scientist at PwC in Bangalore, India.
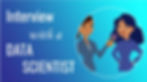
Whether you are thinking of exploring data science as a potential college major or just graduated with a degree in data science, this interview provides some great insights into the profession.
1. Could you give us a general overview of your professional journey? How did you end up in your current field?
I first realized I had an aptitude for math and computer science while studying at Vellore Institute of Technology in TamilNadu, India. Because of this, I looked into the fields of Data Science and Machine Learning, which I learned were the perfect fit for my skills and interests. I deepened my understanding of the field by taking online classes at Data Camp, such as Data Scientist with Python track (https://www.datacamp.com/tracks/data-scientist-with-python). I also participated in hackathons and worked as a Data Scientist Intern with NEC Technology. This hands-on experience allowed me to apply my knowledge to real-world problems.
2. How did you go about deciding what sort of job you wanted?
When searching for a job, I had a clear idea of the type of role I wanted to pursue. I was looking for a position that would challenge me with a heavy focus on data analysis and applications of machine learning and statistics. I also wanted the opportunity to communicate and collaborate with stakeholders to deliver my findings and understand the impact of my solutions on the end client, providing an opportunity for continuous growth and learning.
3. How did you structure your education to prepare you for the job that you wanted?
While pursuing my B.Tech degree, I took courses in linear algebra, calculus and statistics, to understand the mathematical concepts that underlie machine learning. I focused on building a strong foundation in Python programming which is essential in the field of data science. I learned how to implement machine learning models using Python libraries such as sci-kit learn and TensorFlow.
4. How exactly are statistics used in your field? Please describe what aspects of statistics you use the most often.
In my role as a data scientist, I use statistics heavily to understand and analyze data. I utilize statistical concepts such as central tendency, correlation, variance and normalization to clean and analyze data. Hypothesis testing helps me to draw conclusions and make predictions based on data. I also constantly use visualizations such as graphs and plots to help convey information about the data in an easily understandable format.
5. What programming languages do you use the most for your position?
In my current role at PwC, I primarily use Python, because it provides a wide range of libraries and tools that can help manage and analyze data. Python is easy to learn and use, which makes it an excellent choice for beginners.
However, in my previous position at Societe Generale, I worked with R since we built a lot of statistical models. R is specifically designed for statistical computing and graphics, making it an excellent choice for data visualization and exploratory data analysis.
6. Did you need to pick up skills in addition to statistics/computer science for your job?
In addition to a strong foundation in statistics and computer science, I have learned that effective communication, attention to detail, decision-making, and creativity are all essential skills for data scientists. Effective communication is important because we must be able to explain complex concepts and findings to people with different technical backgrounds. Attention to detail is critical because small errors in data or analysis can have significant consequences. We must make calculated decisions based on our analysis and have the creativity to solve problems in innovative ways. Finally, as data scientists, we must be able to present our findings in a clear and concise manner to stakeholders, which requires excellent communication skills. In short, a career in data science requires a combination of technical and soft skills to be successful.
7. What does your daily work routine look like?
As a Consultant Data Scientist, my typical workday starts with a brief call with my project manager to discuss the current status of ongoing projects and identify issues that need to be addressed. After that, I focus on development, testing, tracking, or presentation, depending on the stage of the project. The work varies depending on the project.
8. Are there any particular downsides to your job that you think people should consider before entering the field?
One of the downsides is that dealing with data can be a time-consuming process that requires patience and attention to detail. Rushing through the data cleaning, preparation, modeling and deployment process can result in errors and inaccurate models. Another potential downside is that data science is a relatively new and rapidly evolving field, and many stakeholders may not fully understand the implications of the models that data scientists construct. Moreover, after presenting your results, stakeholders may not always agree with your conclusions, which can be frustrating. In some cases, you may even need to abandon your efforts.
9. What specific advice would you give to students who are thinking of studying statistics/computer science/data science?
The best advice I can give to all students studying data science is to focus on understanding the core concepts and their practical applications. This means developing a strong foundation in mathematics and statistics. It's also important to gain practical experience by working on real-world problems and projects. This can be accomplished through internships or personal projects. Don't get too caught up in pursuing multiple certifications; instead, focus on building a strong portfolio that demonstrates your practical skills and problem-solving abilities.
Takeaway points from Ms. Agarwal's experience:
Developing a strong foundation in mathematics and statistics is crucial for success in data science
Practical experience through internships, hackathons or personal projects is important to gain real world problem solving skills
Effective communication, attention to detail, decision-making, and creativity are all essential skills for data scientists.
Python and R are commonly used programming languages in data science.
Data cleaning and analysis are time-consuming processes that require patience and attention to detail.
It is important to focus on core concepts and their practical applications, rather than pursuing multiple certifications.
I hope this interview has been informative and useful to anyone thinking about a career in data science.